AI In Cybersecurity: Weighing The Pros And Cons
Posted November 1, 2024 by Sayers
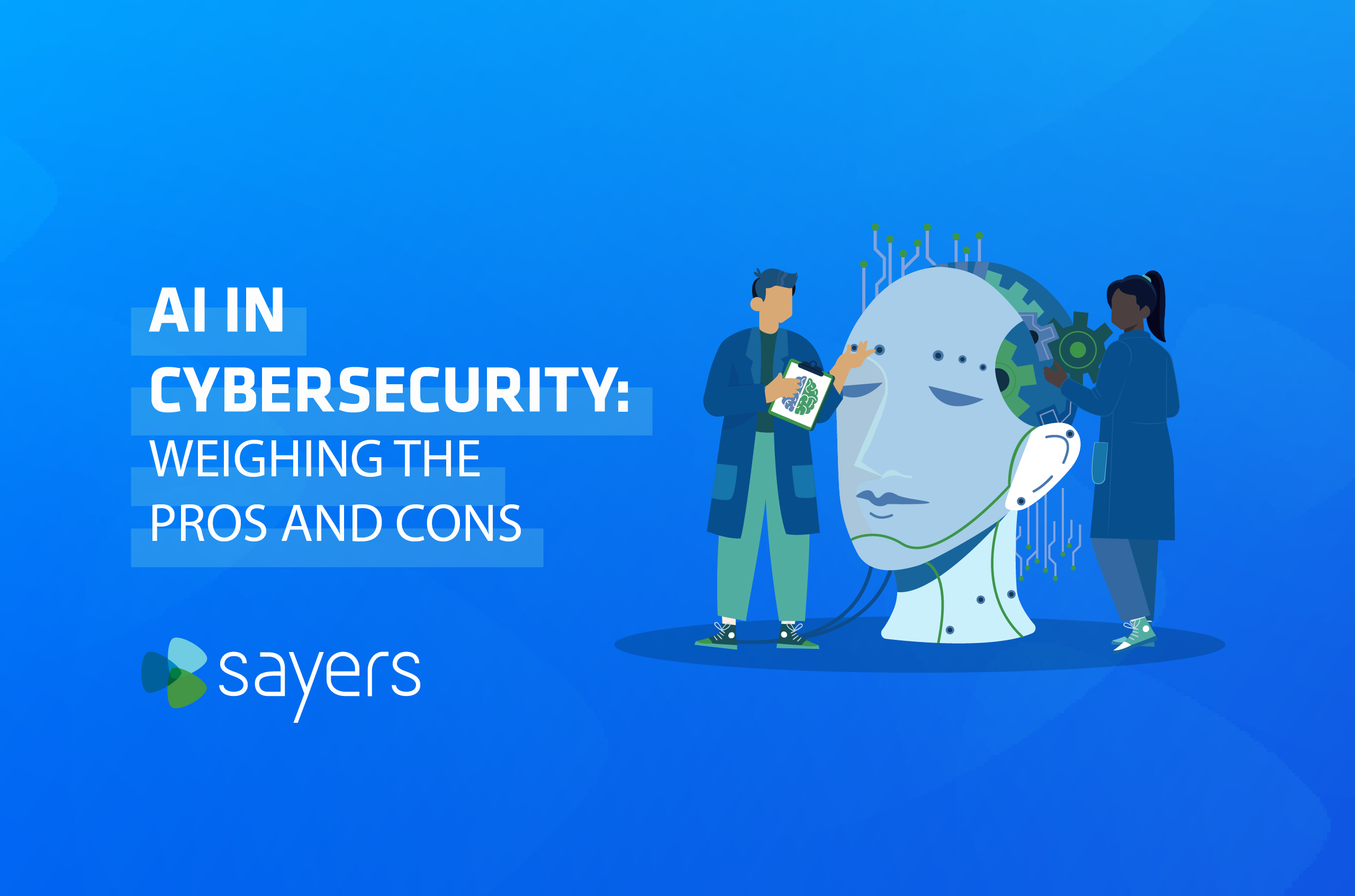
Artificial intelligence has revolutionized industries and brought new capabilities to cybersecurity. As organizations deal with leaner IT and security teams, AI-powered solutions can augment cyber team resources, fill in skills gaps, and automate complex cybersecurity tasks.
But AI’s adoption comes with both advantages and drawbacks, leading to the use and misuse of AI. A growing number of cybersecurity solutions use AI to help organizations protect themselves from attackers, who in turn use AI to hone their attacks.
According to a Palo Alto Networks research report, “The State of Cloud-Native Security”:
61% of organizations fear AI-powered attacks compromise sensitive data. 33% struggle to keep up with rapid technology changes and evolving threats.
The Benefits Of AI In Cybersecurity
Gartner defines AI in cybersecurity as:
“…the application of AI technologies and techniques to enhance the security of computer systems, networks, and data to protect from potential threats and attacks. AI enables cybersecurity systems to analyze vast amounts of data, identify patterns, detect anomalies, and make intelligent decisions in real time to prevent, detect, and respond to cyberthreats.”
Using AI in cybersecurity solutions leads to faster and more accurate threat detection along with greater scalability and cost efficiencies.
1. Faster Threat Detection And Response
When it comes to playing defense against threats, AI is a game-changer. AI-powered cybersecurity solutions analyze enormous amounts of data to identify abnormal behavior and quickly detect malicious activity.
Today’s observability platforms incorporate AI and machine learning to be more intelligent and proactive in monitoring your application, database, infrastructure, and network layers.
AI and machine learning enable predictive analytics, automation, and remediation to unlock a range of capabilities:
- Create an automated performance baseline of your environment
- Proactively detect anomalies
- Alert your team when it detects deviations from that baseline, with minimal false positives
- Improve mean-time-to-recovery of critical assets.
Automation using AI also streamlines security processes such as patch management, enhancing overall cyber readiness.
2. Improved Accuracy And Efficiency
Security automation tools now use AI to quickly analyze massive amounts of data, using AI algorithms to recognize patterns human operators can miss. The result is more accurate threat detection that continues to improve as AI-driven systems learn and adapt.
Kevin Finch, Senior Business Continuity Architect at Sayers, says:
“AI has changed the way we digest data. It does a wonderful job of gathering and summarizing vast volumes of data for concise analysis. That powerful analysis capability is why we see it in so many products coming out these days in the IT world to make that analysis faster, easier, and more accurate.”
AI-powered solutions can scan devices for vulnerabilities significantly faster, saving time and resources. Response time improves as automated systems can react immediately to detected threats.
AI and machine learning are integrating with existing technologies to create hyperautomation capabilities. Hyperautomation goes beyond Security Orchestration, Automation, and Response (SOAR) functionalities to more efficiently and cost-effectively detect, analyze, and respond to security incidents.
3. Greater Scalability And Cost Savings
With AI’s rapid data processing and predictive analytics, you can proactively identify threats without substantial hardware or personnel costs. AI-powered security automation systems can adapt and scale to address potential threats in real time.
AI can update security software across your organization in an instant. By automating those and other routine, tedious security tasks such as network monitoring, AI frees up resources for other business areas or more complex priorities.
Automating labor-intensive cybersecurity tasks reduces security operations costs. For governance and regulations, using AI for more effective detection and response to data breaches helps prevent costly compliance violations.
The Risks Of Relying On AI In Cybersecurity
Counterbalancing the benefits of AI in cybersecurity means recognizing the inherent risks. Your organization should have a plan to address concerns about privacy, vulnerabilities to attacks, and the scope of required resources.
1. Privacy Concerns
AI systems may handle sensitive data, including health and personal user data, which raises privacy and compliance issues. Privacy and data protection legislation have increased in recent years, along with more costly penalties for noncompliance.
Organizations struggle to strike the right balance between security and privacy. The struggle extends to ensuring the privacy of:
- Data used to train and deploy machine learning models.
- Data hosted on external servers, which could pose a security risk for the organization if that data leaks to the public.
- Confidential information entered as prompts into generative AI tools, which could become part of the knowledge base and appear in outputs for other users.
2. Vulnerability To Attacks
Adversarial attacks can manipulate or deceive machine learning models and AI systems, causing them to misinterpret data and leading to dangerous outcomes such as manipulating autonomous vehicles or operational technology systems.
According to Deloitte’s “State of AI in the Enterprise” study:
“Cybersecurity vulnerabilities” tops the list of executives’ concerns about potential AI risks, with 49 percent rating it as a top-three concern.
While technology vendors use AI to create better cybersecurity solutions, bad actors can use it to build more sophisticated malware, phishing attacks, and deepfakes to deceive social engineering attack victims.
Chris Willis, VP of Cybersecurity Engineering at Sayers, says:
“Many cybersecurity vendors are using AI in their solutions to find the bad malware and sophisticated attacks that are happening on the network and through the endpoints. But the adversaries also are using AI to get around those tools. It’s a big battle back and forth.”
AI outputs can be inaccurate in important details, limited with outdated information, and/or skewed due to biased training data. Overreliance on AI may create blind spots, leaving organizations vulnerable to attacks.
3. Resource Intensiveness
Implementing effective AI-based security requires specific resources, including skilled personnel and infrastructure.
GenAI offerings bring adoption and sustainability challenges, such as the increased computing power and cooling needed to process huge volumes of data.
According to Gartner research, GenAI will drive spending in cybersecurity:
Through 2025, generative AI will cause a spike of cybersecurity resources required to secure it, causing more than a 15% incremental spend on application and data security.
What To Consider When Implementing AI In Cybersecurity
Any plan to implement AI in cybersecurity solutions should consider the following to maximize benefits and minimize risks:
1. Data Quality And Quantity
From a quality perspective, the training data for AI models should be accurate, diverse, and representative of real-world threats.
Sufficient data is crucial for robust model performance. Collect enough samples to avoid overfitting, which happens when the machine learning model fits too closely to the training data set and can’t accurately predict outcomes for new data sets.
2. Model Selection And Validation
When choosing machine learning algorithms, such as neural networks or decision trees, be sure they’re based on your specific security use case. Then regularly validate and update models to adapt to evolving threats.
3. Human-AI Collaboration
AI assists humans, but human expertise remains essential. Encourage collaboration between your security analysts and AI systems to keep your organization secure and compliant amid evolving threats and new regulations.
Understand the limitations of AI, such as contextual awareness and perceiving nuance. People must continue interpreting AI’s findings in context.
4. Adversarial Testing
Continuously test AI models against adversarial attacks. Such attacks attempt to manipulate machine learning systems with created inputs that misinterpret data.
Evaluate and train your AI models to make them more robust and resilient. Adversarial training can provide the AI model with examples of adversarial attacks to improve defenses and enhance model security.
Advanced AI security solutions can detect unusual patterns and neutralize adversarial inputs before they can mislead and manipulate the AI model.
5. Interpretable AI
Transparency is key to seeing the AI model’s inner workings and understanding its decision-making. Use interpretable AI models to understand the reasoning behind the model’s decisions.
Examples of interpretable AI models include:
- Decision tree-based, using “if-then” rules to generate predictions
- Linear regression, used for numerical predictions
- Logistic regression, used for classification predictions.
Explainable AI builds trust in the predictive process. A transparent decision-making process also helps organizations demonstrate compliance with laws and regulations.
6. Ethical Considerations
AI can identify human biases but also runs the risk of deploying biases at scale. Prevent discriminatory outcomes by addressing biases in training data. Use a variety of data sources to build in diverse data, and thoroughly test your AI models to identify biased outputs.
Ensure AI systems adhere to ethical guidelines. UNESCO’s most recent recommendations on the Ethics of Artificial Intelligence build on the first-ever global standard on AI ethics previously published in 2021.
Respect user privacy by securing data from unauthorized access and enabling users to control how their data is used.
Questions? Contact us at Sayers today to discover extensive technology solutions, services, and expertise to cover all areas of your business.